Webinars on “Trustworthy Complex and Intelligent Systems”
Webinars will be run monthly throughout 2021 exploring the themes of trust, ethics and applications of AI and novel technology in complex and safety critical intelligent systems.
This series is a collaboration between the European Safety, Reliability & Data Association (external pageESReDAcall_made), the ETH Zürich Chair of Intelligent Maintenance Systems of Risk Center Member prof. Olga Fink, the ETH Risk Center, the Norwegian Research Center for AI Innovation (external pageNorwAIcall_made), external pageDNV GLcall_made, and Risks-X.
Read more external pageherecall_made
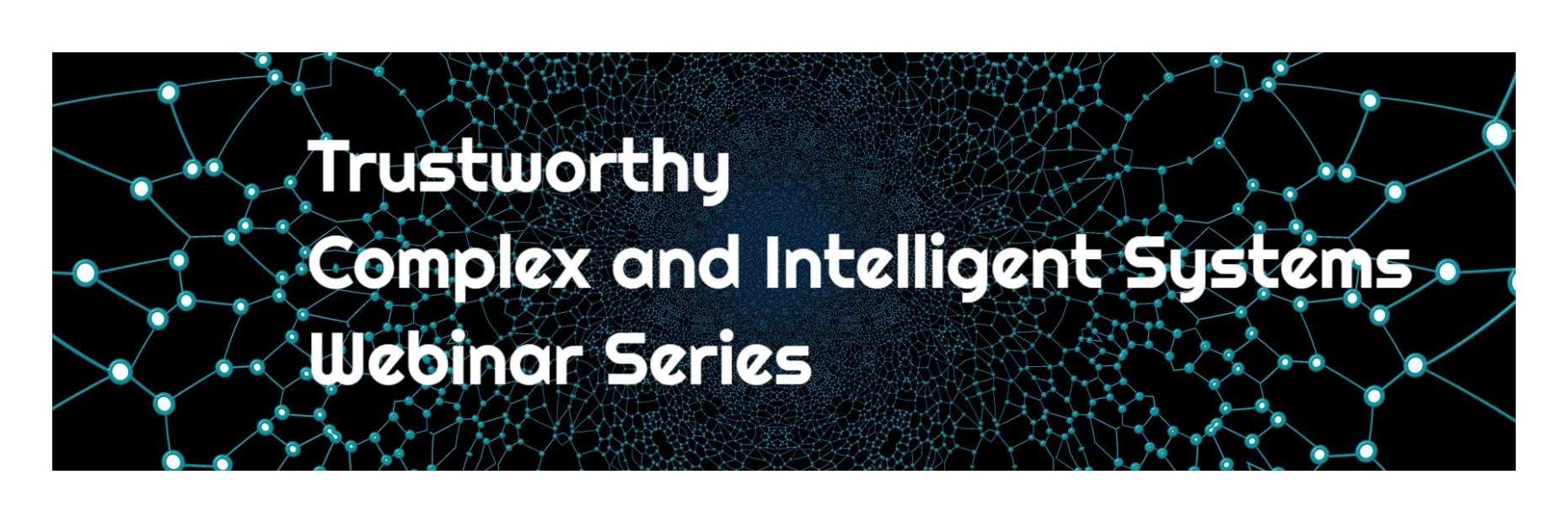
Talk #8: Data-Efficient Deep Learning using Physics-Informed Neural Network
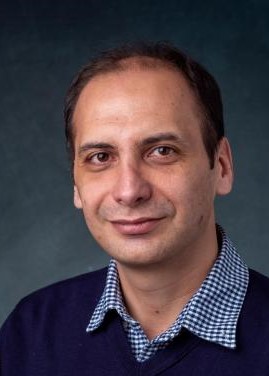
A Webinar with Maziar Raissi, University of Colorado Boulder
A grand challenge with great opportunities is to develop a coherent framework that enables blending conservation laws, physical principles, and/or phenomenological behaviors expressed by differential equations with the vast data sets available in many fields of engineering, science, and technology. At the intersection of probabilistic machine learning, deep learning, and scientific computations, this work is pursuing the overall vision to establish promising new directions for harnessing the long-standing developments of classical methods in applied mathematics and mathematical physics to design learning machines with the ability to operate in complex domains without requiring large quantities of data. To materialize this vision, this work is exploring two complementary directions:
1. designing data-efficient learning machines capable of leveraging the underlying laws of physics, expressed by time dependent and non-linear differential equations, to extract patterns from high-dimensional data generated from experiments, and
2. designing novel numerical algorithms that can seamlessly blend equations and noisy multi-fidelity data, infer latent quantities of interest (e.g., the solution to a differential equation), and naturally quantify uncertainty in computations.
external pageMaziar Raissicall_made is currently an Assistant Professor of Applied Mathematics at the University of Colorado Boulder. Dr. Raissi received a Ph.D. in Applied Mathematics & Statistics, and Scientific Computations from University of Maryland College Park. He moved to Brown University to carry out postdoctoral research in the Division of Applied Mathematics. Dr. Raissi worked at NVIDIA in Silicon Valley for a little more than one year as a Senior Software Engineer before moving to Boulder. His expertise lies at the intersection of Probabilistic Machine Learning, Deep Learning, and Data Driven Scientific Computing. In particular, he has been actively involved in the design of learning machines that leverage the underlying physical laws and/or governing equations to extract patterns from high-dimensional data generated from experiments.
Watch the replay external pageherecall_made
Read the slides external pageherecall_made
Talk #7: The Strange Case of Dr Trust and Mr Interpretability in Human-AI Interactions
A Webinar with Andrea Ferrario, ETH Zurich
The terms "trust", "interpretability" and their cognates are commonly used to describe situations where humans interact with artificial intelligence (AI) systems. From healthcare to insurance applications, fostering trust is usually considered as a possible goal of the interaction itself, while interpretability of AI predictions and their "inner working" is seen as a way to achieve it. Unfortunately, researchers do not converge to a common definition of these terms, with the effect of devaluing scientific results from the explainable AI research domain.
Andrea Ferrario holds a PhD in Mathematics from ETH Zürich. After this PhD he spent 5+ years in consulting, specializing in data & analytics. Since 2018, he is back at ETH Zürich as a PostDoc in the Chair of Technology Marketing at ETH MTEC, and the Scientific Director of the Mobiliar Lab for Analytics at ETH Zürich. His research interests lie at the intersection between philosophy and technology, with a focus on the epistemological and ethical problems of AI, and the use of machine learning and immersive analytics for healthcare, insurance and higher education applications.
Talk #6: Prognostics and Health Management for Condition-based and Predictive Maintenance: A Look In and a Look Out
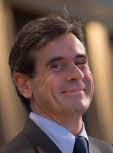
A Webinar with Enrico Zio, CRC MINES ParisTech, France & Politecnico di Milano, Italy
A number of methods of Prognostics and Health Management (PHM) have been developed (and more are being developed) for use in diverse engineering applications. Yet, there are still a number of critical problems which impede full deployment of PHM and its benefits in practice. In this lecture, we look in some of these PHM challenges and look out to advancements for PHM deployment.
external pageEnrico Ziocall_made is full professor at the Centre for research on Risk and Crises (CRC) of Ecole de Mines, ParisTech, PSL University, France, full professor and President of the Alumni Association at Politecnico di Milano, Italy. His research focuses on the modeling of the failure-repair-maintenance behavior of components and complex systems, for the analysis of their reliability, maintainability, prognostics, safety, vulnerability, resilience and security characteristics, and on the development and use of Monte Carlo simulation methods, artificial intelligence techniques and optimization heuristics. In 2020, he has been awarded the prestigious Humboldt Research Award from the Alexander von Humboldt Foundation in Germany.
Watch the replay external pageherecall_made
Talk #5: Zeabuz: Providing Trust in a Zero Emission Autonomous Passenger Ferry
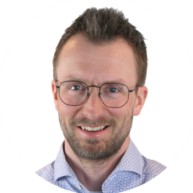
A Webinar with Øyvind Smogeli, CTO Zeabuz
This talk introduces the Zeabuz mobility concept, the autonomy architecture, then will focus on the many layers of trust and how to achieve this. The various components of the autonomy system and the simulation technology used to build trust in the autonomy are explained. An approach to build trust in the simulators through field experiments and regular operation will be presented. It will be shown how this all fits into the larger assurance case.
external pageØyvind Smogelicall_made is the CTO and co-founder of Zeabuz and an Adjunct Professor at NTNU. Øyvind received his PhD from NTNU in 2006, and has spent his career working on modeling, simulation, testing and verification, complex cyber-physical systems, and assurance of digital technologies. He has previously held positions as CTO, COO and CEO of Marine Cybernetics and as Research Program Director for Digital Assurance in DNV.
Watch the replay external pageherecall_made
Talk #4: Certified Deep Learning
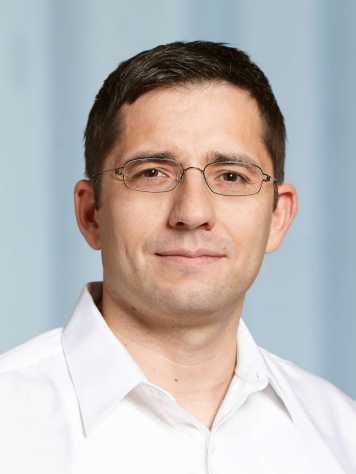
A Webinar with Martin Vechev (ETH Zurich SRILab).
In this talk I will discuss some of the latest progress we have made in the space of certifying AI systems, ranging from certification of deep neural networks to entire deep learning pipelines. In the process I will also discuss new neural architectures that are more amenable to certification as well as mathematical impossibility and complexity results that help guide new kinds of certified training methods.
Martin Vechev is an Associate Professor at the Department of Computer Science, ETH Zurich. His work spans the intersection of machine learning and symbolic methods with applications to topics such as safety of artificial intelligence, quantum programming and security. He has co-founded 3 start-ups in the space of AI and security, the latest of which LatticeFlow aims to build and deploy trustworthy AI models.
Watch the replay external pageherecall_made
Talk #2: Structured models of physics, objects, and scenes
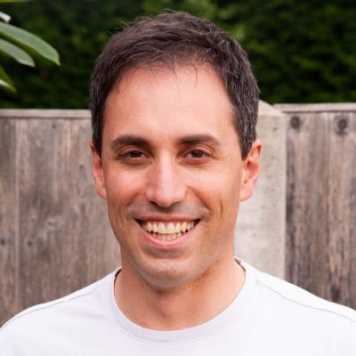
A Webinar with Peter Battaglia (Deep Mind).
This talk will describe various ways of using structured machine learning models for predicting complex physical dynamics, generating realistic objects, and constructing physical scenes. The key insight is that many systems can be represented as graphs with nodes connected by edges, which can be processed by graph neural networks and transformer-based models. By considering the underlying structure of the problem, and imposing inductive biases within our models that reflect them, we can often achieve more accurate, efficient, and generalizable performance than if we avoid using principled assumptions.
Peter Battaglia is a research scientist at DeepMind working on approaches for reasoning about and interacting with complex systems.
Watch the replay external pageherecall_made
Talk #1: Why is it so hard to make self-driving cars?
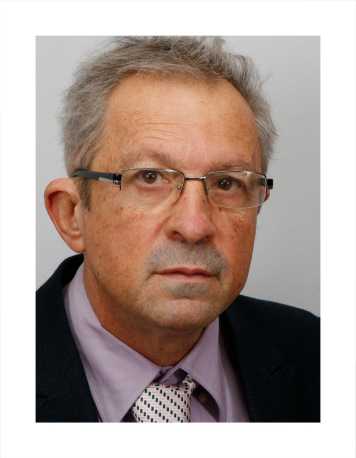
A Webinar with Joseph Sifakis.
Why is the problem of self-driving autonomous control so hard? Despite the enthusiastic involvement of big technological companies and investment of billions of dollars, optimistic predictions about the realization of autonomous vehicles have yet to materialize.
Hear 2007 Turing Award winner Joseph Sifakis explain the challenges raised by the vision for trustworthy autonomous systems for the autonomous vehicle case and outline his hybrid design approach combining model-based and data-based techniques and seeking trade offs between performance and trustworthiness.
Watch the replay external pageherecall_made